
What is Data Quality Management?
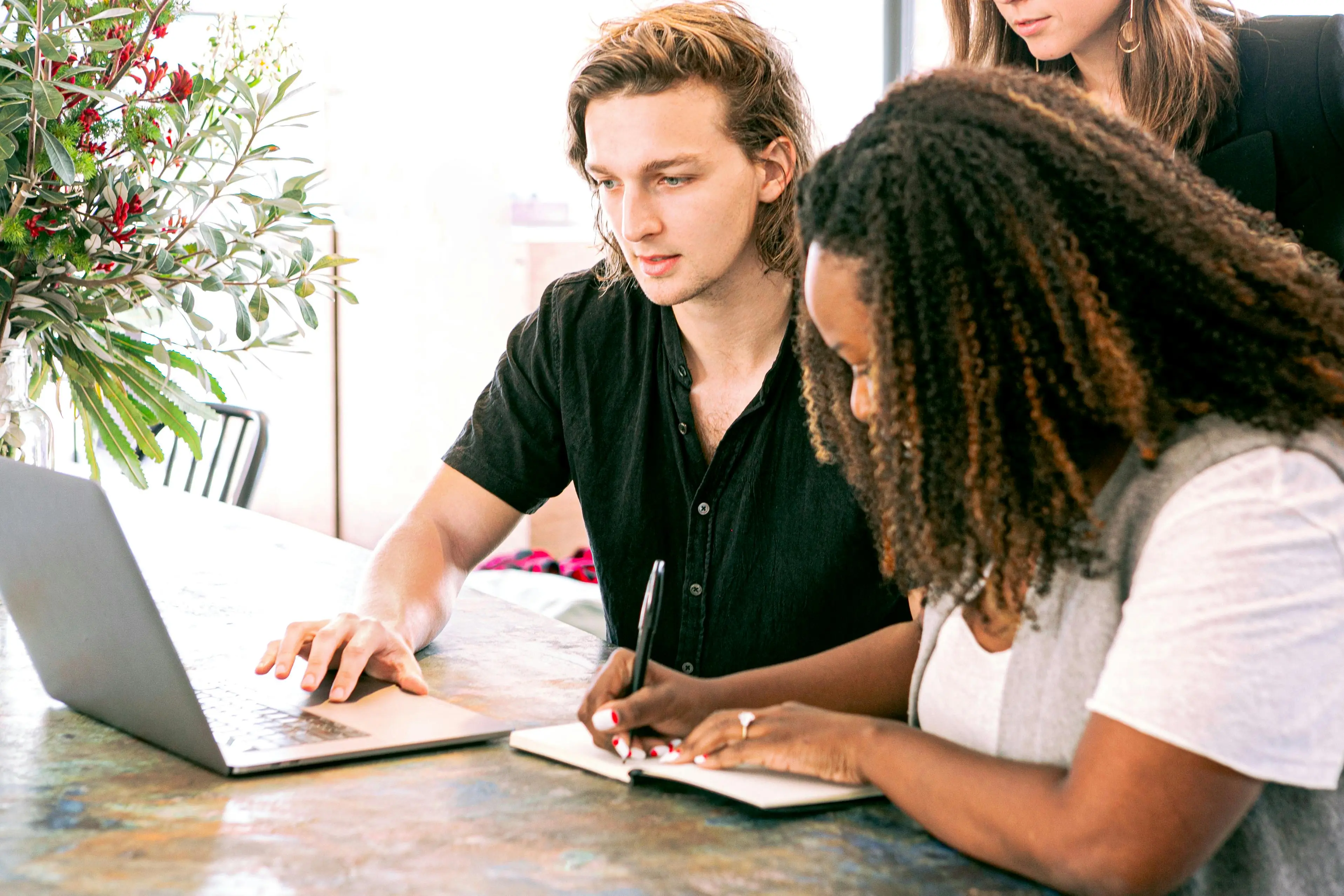
In today's data-driven world, data quality is essential for any organization. Data Quality Management (DQM) is a crucial practice that ensures data is accurate, consistent and reliable. But what exactly is Data Quality Management, and why is it so important?
Data Quality Management is a set of practices and processes designed to ensure that an organization's data meets high quality standards. It involves the assessment, monitoring and continuous improvement of data accuracy, completeness, consistency and reliability.
The primary objective of DQM is to ensure that data is reliable and appropriate for its intended use, whether for decision-making, analysis or regulatory compliance.
What are the data quality issues?
Data quality problems can have a significant impact on a company's operations and reputation. Here are just a few common problems:
- Missing or incomplete data: empty fields or missing information can affect analysis and decision-making.
- Data entry errors: human errors can lead to inconsistencies, duplications or incorrect information.
- Redundant data: duplicate data can lead to inconsistencies and inefficient use of resources.
- Obsolete data: obsolete information can lead to erroneous decisions and affect the accuracy of analyses.
- Inconsistencies: inconsistent data formats or standards can make data difficult to interpret and use.
Data Quality tools
A variety of software solutions are available to effectively manage data quality. These tools help automate and streamline DQM processes:
- Data profiling tools: analyze data to provide information on its structure, content and quality.
- Data cleansing solutions: these tools help identify and correct errors, duplicates and inconsistencies.
- Data management software: these solutions provide a centralized view of data, ensuring consistency and accuracy.
- Data quality monitoring platforms: these tools continuously monitor data quality and alert you to any problems.
- Data profiling tools: analyze data to provide information on its structure, content and quality.
- Data cleansing solutions: these tools help identify and correct errors, duplicates and inconsistencies.
- Data management software: these solutions provide a centralized view of data, ensuring consistency and accuracy.
- Data quality monitoring platforms: these tools continuously monitor data quality and alert you to any problems.
Key roles in Data Quality Management
Data Quality Manager
The Data Quality Manager is responsible for the overall supervision of DQM practices within an organization. Key missions include :
- Define data quality standards and policies
- Oversee the implementation of DQM processes
- Manage DQM tools and technologies
- Train and raise employee awareness of data quality best practices
- Collaborate with other departments to ensure alignment of DQM practices with organizational objectives
Data Quality Analyst
The Data Quality Analyst is responsible for executing DQM processes on a day-to-day basis. Key roles include:
- Analyze and assess data quality
- Identify data quality problems and propose solutions
- Implement data cleansing and standardization processes
- Monitor and report on data quality indicators
- Collaborate with IT and business teams to resolve data quality issues
What are Data Quality indicators?
Data Quality Indicators (KPIs) are essential for assessing the effectiveness of DQM practices. Here are some common KPIs:
- Accuracy: percentage of correct and accurate data.
- Completeness: measurement of the rate of missing or incomplete data.
- Consistency: assessment of data consistency across different systems or sources.
- Uniqueness: percentage of unique, unduplicated data.
- Timeliness: a measure of the timeliness of data in relation to its intended use.
Data Quality and software PIM
Product information management (PIM) software plays a crucial role in DQM, especially for companies with a large number of products and associated data. PIM ensures that product data is centralized, standardized and efficiently managed. It improves data quality by :
- Providing a single source of truth for product data
- Automating data cleansing and standardization
- Enabling efficient collaboration between teams
- Offering data validation and quality control functionalities
In conclusion, Data Quality Management is essential to maintaining the integrity and reliability of data within an organization. By investing in the right tools, the right people and the right practices, companies can ensure that their data is valuable and contributes to their success.
Interested in Data Quality and PIM ? Request your free demo!